
Evaluation measures (information retrieval)
Evaluation measures for an information retrieval (IR) system assess how well an index, search engine, or database returns results from a collection of resources that satisfy a user's query. They are therefore fundamental to the success of information systems and digital platforms.

The most important factor in determining a system's effectiveness for users is the overall relevance of results retrieved in response to a query.[1] The success of an IR system may be judged by a range of criteria including relevance, speed, user satisfaction, usability, efficiency and reliability.[2] Evaluation measures may be categorised in various ways including offline or online, user-based or system-based and include methods such as observed user behaviour, test collections, precision and recall, and scores from prepared benchmark test sets.

Evaluation for an information retrieval system should also include a validation of the measures used, i.e. an assessment of how well they measure what they are intended to measure and how well the system fits its intended use case.[3] Measures are generally used in two settings: online experimentation, which assesses users' interactions with the search system, and offline evaluation, which measures the effectiveness of an information retrieval system on a static offline collection.

Background
Indexing and classification methods to assist with information retrieval have a long history dating back to the earliest libraries and collections however systematic evaluation of their effectiveness began in earnest in the 1950s with the rapid expansion in research production across military, government and education and the introduction of computerised catalogues. At this time there were a number of different indexing, classification and cataloguing systems in operation which were expensive to produce and it was unclear which was the most effective.[4]

Cyril Cleverdon, Librarian of the College of Aeronautics, Cranfield, England, began a series of experiments of print indexing and retrieval methods in what is known as the Cranfield paradigm, or Cranfield tests, which set the standard for IR evaluation measures for many years.[4] Cleverdon developed a test called ‘known-item searching’ - to check whether an IR system returned the documents that were known to be relevant or correct for a given search. Cleverdon’s experiments established a number of key aspects required for IR evaluation: a test collection, a set of queries and a set of pre-determined relevant items which combined would determine precision and recall.

Cleverdon's approach formed a blueprint for the successful Text Retrieval Conference series that began in 1992.

Applications
Evaluation of IR systems is central to the success of any search engine including internet search, website search, databases and library catalogues. Evaluations measures are used in studies of information behaviour, usability testing, business costs and efficiency assessments. Measuring the effectiveness of IR systems has been the main focus of IR research, based on test collections combined with evaluation measures.[5] A number of academic conferences have been established that focus specifically on evaluation measures including the Text Retrieval Conference (TREC), Conference and Labs of the Evaluation Forum (CLEF) and NTCIR.

Online measures
Online metrics are generally created from search logs. The metrics are often used to determine the success of an A/B test.

Session abandonment rate
Session abandonment rate is a ratio of search sessions which do not result in a click.

Click-through rate
Click-through rate (CTR) is the ratio of users who click on a specific link to the number of total users who view a page, email, or advertisement. It is commonly used to measure the success of an online advertising campaign for a particular website as well as the effectiveness of email campaigns.[6]

Session success rate
Session success rate measures the ratio of user sessions that lead to a success. Defining "success" is often dependent on context, but for search a successful result is often measured using dwell time as a primary factor along with secondary user interaction, for instance, the user copying the result URL is considered a successful result, as is copy/pasting from the snippet.

Zero result rate
Zero result rate (ZRR) is the ratio of Search Engine Results Pages (SERPs) which returned with zero results. The metric either indicates a recall issue, or that the information being searched for is not in the index.
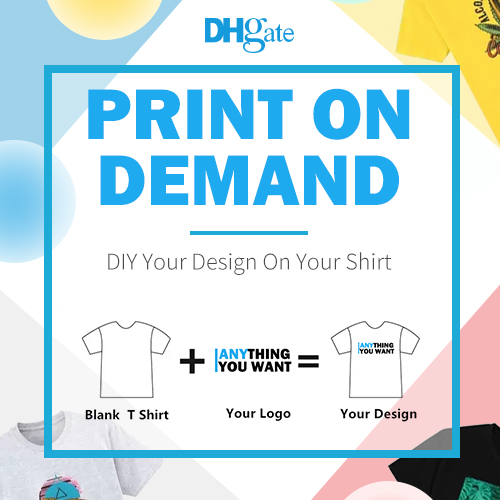
Offline metrics
Offline metrics are generally created from relevance judgment sessions where the judges score the quality of the search results. Both binary (relevant/non-relevant) and multi-level (e.g., relevance from 0 to 5) scales can be used to score each document returned in response to a query. In practice, queries may be ill-posed, and there may be different shades of relevance. For instance, there is ambiguity in the query "mars": the judge does not know if the user is searching for the planet Mars, the Mars chocolate bar, the singer Bruno Mars, or the Roman deity Mars.

Precision
Precision is the fraction of the documents retrieved that are relevant to the user's information need.

In binary classification, precision is analogous to positive predictive value. Precision takes all retrieved documents into account. It can also be evaluated considering only the topmost results returned by the system using Precision@k.

Note that the meaning and usage of "precision" in the field of information retrieval differs from the definition of accuracy and precision within other branches of science and statistics.

Recall
Recall is the fraction of the documents that are relevant to the query that are successfully retrieved.

In binary classification, recall is often called sensitivity. So it can be looked at as the probability that a relevant document is retrieved by the query.

It is trivial to achieve recall of 100% by returning all documents in response to any query. Therefore, recall alone is not enough but one needs to measure the number of non-relevant documents also, for example by computing the precision.

Fall-out
The proportion of non-relevant documents that are retrieved, out of all non-relevant documents available:

In binary classification, fall-out is the opposite of specificity and is equal to . It can be looked at as the probability that a non-relevant document is retrieved by the query.

It is trivial to achieve fall-out of 0% by returning zero documents in response to any query.

F-score / F-measure
The weighted harmonic mean of precision and recall, the traditional F-measure or balanced F-score is:

This is also known as the measure, because recall and precision are evenly weighted.

The general formula for non-negative real is:

Two other commonly used F measures are the measure, which weights recall twice as much as precision, and the measure, which weights precision twice as much as recall.

The F-measure was derived by van Rijsbergen (1979) so that "measures the effectiveness of retrieval with respect to a user who attaches times as much importance to recall as precision". It is based on van Rijsbergen's effectiveness measure . Their relationship is:

- where
Since F-measure combines information from both precision and recall it is a way to represent overall performance without presenting two numbers.

Average precision
Precision and recall are single-value metrics based on the whole list of documents returned by the system. For systems that return a ranked sequence of documents, it is desirable to also consider the order in which the returned documents are presented. By computing a precision and recall at every position in the ranked sequence of documents, one can plot a precision-recall curve, plotting precision as a function of recall . Average precision computes the average value of over the interval from to :[7]

That is the area under the precision-recall curve. This integral is in practice replaced with a finite sum over every position in the ranked sequence of documents:

where is the rank in the sequence of retrieved documents, is the number of retrieved documents, is the precision at cut-off in the list, and is the change in recall from items to .[7]

This finite sum is equivalent to:

where is an indicator function equaling 1 if the item at rank is a relevant document, zero otherwise.[8] Note that the average is over relevant documents in top-k retrieved documents and the relevant documents not retrieved get a precision score of zero.

Some authors choose to interpolate the function to reduce the impact of "wiggles" in the curve.[9][10] For example, the PASCAL Visual Object Classes challenge (a benchmark for computer vision object detection) until 2010[11] computed the average precision by averaging the precision over a set of evenly spaced recall levels {0, 0.1, 0.2, ... 1.0}:[9][10]

where is an interpolated precision that takes the maximum precision over all recalls greater than :
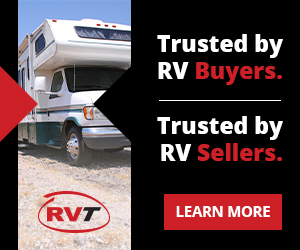
- .
An alternative is to derive an analytical function by assuming a particular parametric distribution for the underlying decision values. For example, a binormal precision-recall curve can be obtained by assuming decision values in both classes to follow a Gaussian distribution.[12]

The minimum achievable AveP for a given classification task is given by:


Precision at k
For modern (web-scale) information retrieval, recall is no longer a meaningful metric, as many queries have thousands of relevant documents, and few users will be interested in reading all of them. Precision at k documents (P@k) is still a useful metric (e.g., P@10 or "Precision at 10" corresponds to the number of relevant results among the top 10 retrieved documents), but fails to take into account the positions of the relevant documents among the top k.[14] Another shortcoming is that on a query with fewer relevant results than k, even a perfect system will have a score less than 1.[15] It is easier to score manually since only the top k results need to be examined to determine if they are relevant or not.

R-precision
R-precision requires knowing all documents that are relevant to a query. The number of relevant documents, , is used as the cutoff for calculation, and this varies from query to query. For example, if there are 15 documents relevant to "red" in a corpus (R=15), R-precision for "red" looks at the top 15 documents returned, counts the number that are relevant turns that into a relevancy fraction: .[16]

Note that the R-Precision is equivalent to both the precision at the -th position (P@) and the recall at the -th position.[15]

Empirically, this measure is often highly correlated to mean average precision.[15]

Mean average precision
Mean average precision (MAP) for a set of queries is the mean of the average precision scores for each query.

where Q is the number of queries.

Discounted cumulative gain
DCG uses a graded relevance scale of documents from the result set to evaluate the usefulness, or gain, of a document based on its position in the result list. The premise of DCG is that highly relevant documents appearing lower in a search result list should be penalized as the graded relevance value is reduced logarithmically proportional to the position of the result.

The DCG accumulated at a particular rank position is defined as:

Since result set may vary in size among different queries or systems, to compare performances the normalised version of DCG uses an ideal DCG. To this end, it sorts documents of a result list by relevance, producing an ideal DCG at position p (), which normalizes the score:

The nDCG values for all queries can be averaged to obtain a measure of the average performance of a ranking algorithm. Note that in a perfect ranking algorithm, the will be the same as the producing an nDCG of 1.0. All nDCG calculations are then relative values on the interval 0.0 to 1.0 and so are cross-query comparable.

Other measures
- Mean reciprocal rank
- bpref - a summation-based measure of how many relevant documents are ranked before irrelevant documents[16]
- GMAP - geometric mean of (per-topic) average precision[16]
- Measures based on marginal relevance and document diversity - see Relevance (information retrieval) § Problems and alternatives
- Measures of both relevance and credibility (for fake news in search results)[17]
- Hit Rate
Visualization
Visualizations of information retrieval performance include:

- Graphs which chart precision on one axis and recall on the other[16]
- Histograms of average precision over various topics[16]
- Receiver operating characteristic (ROC curve)
- Confusion matrix
Non-relevance measures
Queries per time
Measuring how many queries are performed on the search system per (month/day/hour/minute/sec) tracks the utilization of the search system. It can be used for diagnostics to indicate an unexpected spike in queries, or simply as a baseline when comparing with other metrics, like query latency. For example, a spike in query traffic, may be used to explain a spike in query latency.

See also
References
- ^ Carterette, Ben; Voorhees, Ellen M. (2011), Lupu, Mihai; Mayer, Katja; Tait, John; Trippe, Anthony J. (eds.), "Overview of Information Retrieval Evaluation", Current Challenges in Patent Information Retrieval, Berlin, Heidelberg: Springer, pp. 69–85, doi:10.1007/978-3-642-19231-9_3, ISBN 978-3-642-19231-9, retrieved 2022-12-09
- ^ Clough, P.; Sanderson, M. (2013-06-15). "Evaluating the performance of information retrieval systems using test collections". Information Research. Retrieved 2022-12-09.
- ^ Karlgren, Jussi (2019). "Adopting systematic evaluation benchmarks in operational settings" (PDF). Information Retrieval in a Changing World. Retrieved 27 June 2022.
- ^ a b Harman, Donna (2011). Information Retrieval Evaluation. Synthesis Lectures on Information Concepts, Retrieval, and Services. Cham, Switzerland: Springer. doi:10.1007/978-3-031-02276-0. ISBN 978-3-031-02276-0. S2CID 207318946.
- ^ Sanderson, Mark (2010). "Test Collection Based Evaluation of Information Retrieval Systems". Foundations and Trends in Information Retrieval. 4 (4): 247–375. doi:10.1561/1500000009. ISSN 1554-0669.
- ^ American Marketing Association Dictionary. [1] Retrieved 2012-11-02. The Marketing Accountability Standards Board (MASB) endorses this definition as part of its ongoing Common Language in Marketing Project Archived 2019-04-05 at the Wayback Machine.
- ^ a b Zhu, Mu (2004). "Recall, Precision and Average Precision" (PDF). Archived from the original (PDF) on 2011-05-04.
{{cite journal}}
: Cite journal requires|journal=
(help) - ^ Turpin, Andrew; Scholer, Falk (2006). "User performance versus precision measures for simple search tasks". Proceedings of the 29th annual international ACM SIGIR conference on Research and development in information retrieval. New York, NY: ACM. pp. 11–18. CiteSeerX 10.1.1.533.4100. doi:10.1145/1148170.1148176. ISBN 978-1-59593-369-0. S2CID 9810253.
- ^ a b Everingham, Mark; Van Gool, Luc; Williams, Christopher K. I.; Winn, John; Zisserman, Andrew (June 2010). "The PASCAL Visual Object Classes (VOC) Challenge" (PDF). International Journal of Computer Vision. 88 (2): 303–338. doi:10.1007/s11263-009-0275-4. hdl:20.500.11820/88a29de3-6220-442b-ab2d-284210cf72d6. S2CID 4246903. Archived from the original (PDF) on 2011-11-20. Retrieved 2011-08-29.
- ^ a b Manning, Christopher D.; Raghavan, Prabhakar; Schütze, Hinrich (2008). Introduction to Information Retrieval. Cambridge University Press.
- ^ "The PASCAL Visual Object Classes Challenge 2012 (VOC2012) Development Kit". host.robots.ox.ac.uk. Retrieved 2019-03-23.
- ^ K.H. Brodersen, C.S. Ong, K.E. Stephan, J.M. Buhmann (2010). The binormal assumption on precision-recall curves Archived December 8, 2012, at the Wayback Machine. Proceedings of the 20th International Conference on Pattern Recognition, 4263-4266.
- ^ Boyd, K., Davis, J., Page, D., & Costa, V.S. (2012). Unachievable Region in Precision-Recall Space and Its Effect on Empirical Evaluation. Proceedings of the ... International Conference on Machine Learning. International Conference on Machine Learning, 2012, 349 .
- ^ Kalervo, J~irvelin (2017). "IR evaluation methods for retrieving highly relevant documents" (PDF). ACM SIGIR Forum. 51, 2: 243–250.
- ^ a b c Christopher D. Manning; Prabhakar Raghavan & Hinrich Schütze (2009). "Chapter 8: Evaluation in information retrieval" (PDF). Retrieved 2015-06-14. Part of Introduction to Information Retrieval [2]
- ^ a b c d e http://trec.nist.gov/pubs/trec15/appendices/CE.MEASURES06.pdf [bare URL PDF]
- ^ C. Lioma; J. G. Simonsen; B. Larsen (2017). "Evaluation Measures for Relevance and Credibility in Ranked Lists" (PDF). Proceedings of the ACM SIGIR International Conference on Theory of Information Retrieval, 91-98.
See what we do next...
OR
By submitting your email or phone number, you're giving mschf permission to send you email and/or recurring marketing texts. Data rates may apply. Text stop to cancel, help for help.
Success: You're subscribed now !